Enhancing Manufacturing Efficiency with Generative AI and Predictive Maintenance
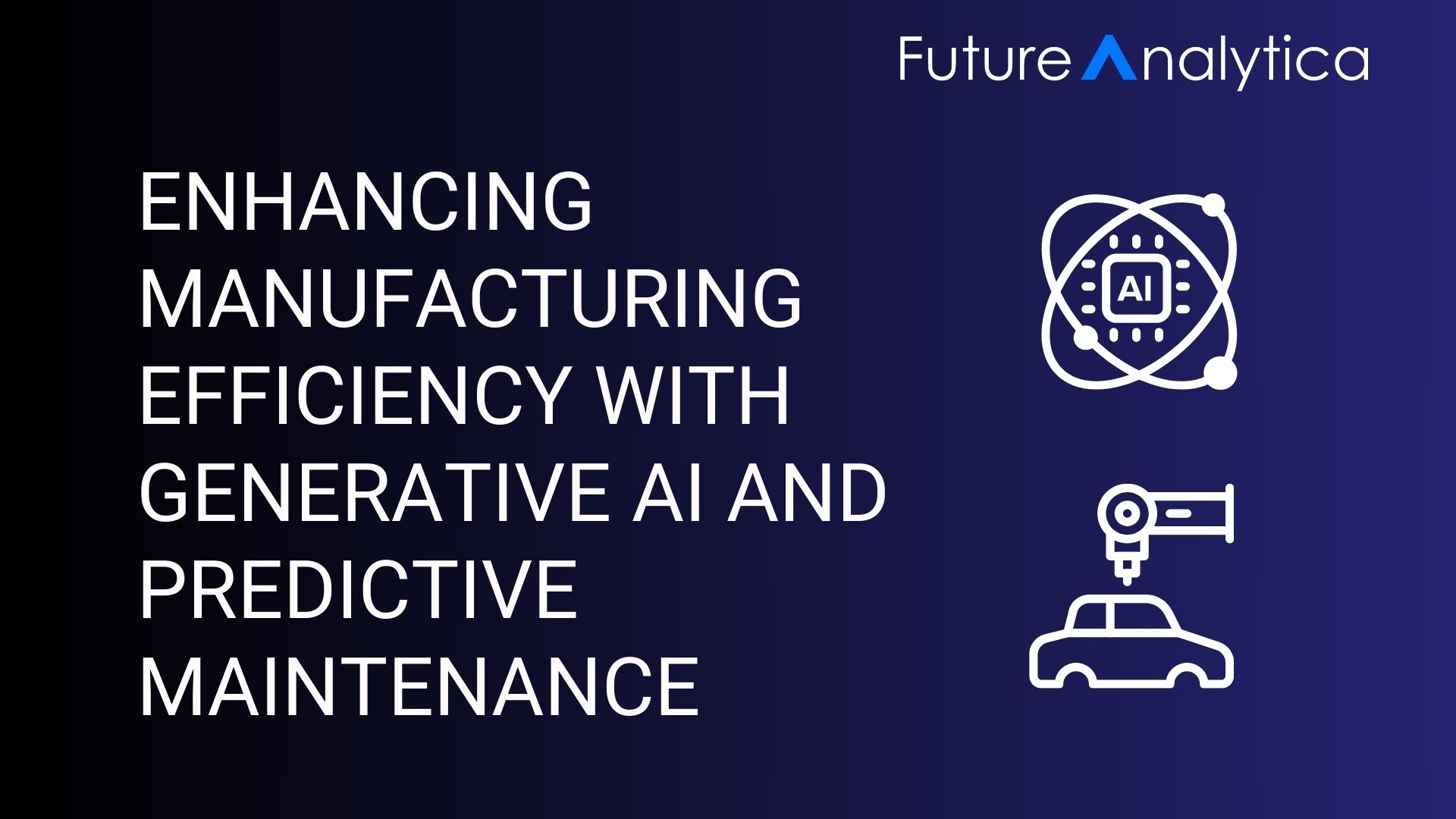
In the fast-paced world of manufacturing, every minute counts. Downtime, whether planned or unplanned, can have significant financial implications. Understanding the true cost of downtime and leveraging advanced technologies like Generative AI for anomaly detection can help manufacturers minimize disruptions and optimize their operations. This blog post delves into the cost of downtime in manufacturing and explores how Generative AI can be a game-changer in anomaly detection.
Understanding Downtime in Manufacturing
Downtime in manufacturing refers to any period when production is halted. It can be categorized into planned and unplanned downtime. Planned downtime includes scheduled maintenance, equipment upgrades, and other activities that are intentionally timed to minimize disruption. Unplanned downtime, on the other hand, occurs unexpectedly due to equipment failures, process breakdowns, or human errors.
The Financial Impact of Downtime
The financial impact of downtime is staggering. According to a study by Aberdeen Research, unplanned downtime can cost a manufacturing company as much as $260,000 per hour. This figure includes direct costs such as lost production value and repair expenses, as well as indirect costs like labor expenses for idle workers, missed deadlines, and reputational damage.
A report by Siemens highlights that the cost of unplanned downtime has increased significantly over the past few years, with Fortune Global 500 companies losing approximately 11% of their yearly turnover due to downtime, amounting to nearly $1.5 trillion. This increase is attributed to factors such as inflation, higher production capacities, and more complex supply chains.
Calculating the Cost of Downtime
Calculating the cost of downtime involves several factors. A generalized formula for downtime cost is:
$$ \text{Downtime Cost} = (\text{Duration of Downtime} \times \text{Cost per Minute of Downtime}) + \text{Lost Revenue} + \text{Cost of Overtime} $$
Here, direct costs include lost production value, wasted raw materials, and repair costs. Indirect costs comprise lost sales opportunities, overtime labor costs, and damage to brand reputation
The Role of Generative AI in Anomaly Detection
Generative AI, powered by deep learning models such as Generative Adversarial Networks (GANs) and Variational Autoencoders (VAEs), has emerged as a powerful tool for anomaly detection. Its ability to generate data that closely resembles a given dataset makes it particularly effective in identifying anomalies.
How Generative AI Works for Anomaly Detection
- Training the Model: The Generative AI model is trained on a dataset containing normal, non-anomalous data. This enables the model to learn the patterns and features of “normal” data.
- Generating Synthetic Data: Once trained, the model can generate synthetic data that closely resembles the normal data it learned from. This synthetic data serves as a reference point for what “normal” data should look like.
- Comparing Real Data to Synthetic Data: When new data is introduced for anomaly detection, it is compared to the synthetic data. If the real data significantly deviates from the synthetic data distribution, it is flagged as an anomaly.
- Adaptive Learning: Generative AI models can adapt and improve over time. As more data becomes available, the model can fine-tune its understanding of normal data, making it even more effective at detecting anomalies
Benefits of Generative AI for Anomaly Detection
- Unsupervised Learning: Generative AI operates in an unsupervised manner, meaning it doesn’t require labeled anomaly data for training. This makes it highly adaptable to various use cases.
- Early Detection: By learning the nuances of normal data, Generative AI can detect anomalies at an early stage, potentially preventing costly issues or seizing opportunities before they escalate.
- Reduced False Positives: Generative AI tends to produce fewer false positive alerts, as it focuses on the unique characteristics of normal data rather than predefined thresholds.
- Continuous Improvement: With continuous data updates, Generative AI models can adapt and improve, staying relevant and effective in dynamic environments
Case Study: Predictive Maintenance in Automotive Manufacturing
One of our clients, a leading automotive manufacturer, faced challenges associated with unplanned downtime and maintenance costs, impacting production efficiency and profitability. By implementing a predictive maintenance strategy leveraging advanced machine learning techniques, we were able to address these challenges effectively
Data Collection and Preprocessing
The first step involved collecting data from various sensors installed on critical machinery and equipment throughout the manufacturing plant. This data included sensor readings such as temperature, vibration, and pressure of chain wear links. The raw sensor data was pre-processed to remove noise, handle missing values, and normalize features.
Model Development and Training
A Generative Deep Learning architecture was developed to serve as an encoder for anomaly detection in the sensor data. The deep learning network learned to reconstruct normal sensor readings accurately, while the error in reconstruction correlated with the anomaly. This information was then projected for machine learning algorithms to learn the patterns, feature impact, and effects to gain deep insights into anomalies.
Results and Impact
The implementation of anomaly analytics using Generative AI and Machine Learning for predictive maintenance yielded significant benefits for the automotive manufacturer. The model demonstrated high accuracy in detecting anomalies and predicting chain link failures well in advance. As a result, the client experienced reduced downtime, lower maintenance costs, and improved overall equipment effectiveness (OEE). Production efficiency and product quality were enhanced, leading to increased customer satisfaction and profitability
In summary, the results were remarkable:
- High Detection Accuracy: The AI model achieved a 97% F1 score in anomaly detection, significantly reducing false positives and negatives.
- Reduced Downtime: Proactive maintenance based on predictive insights led to a substantial decrease in unplanned downtime.
- Cost Savings: The company experienced lower maintenance costs and improved overall equipment effectiveness (OEE).
Challenges and Considerations
While Generative AI holds immense promise for anomaly detection, it is not without its challenges. Ensuring data privacy and security, model interpretability, and the need for substantial computational resources are some of the considerations organizations must address when implementing this technology
Conclusion
Downtime in manufacturing is a costly issue that can significantly impact a company’s bottom line. Understanding the true cost of downtime and leveraging advanced technologies like Generative AI for anomaly detection can help manufacturers minimize disruptions and optimize their operations. At Future Analytica, we are committed to harnessing the power of Generative AI and Machine Learning to provide innovative solutions for anomaly detection in manufacturing. By proactively addressing equipment failures and optimizing production processes, we help our clients achieve significant operational efficiencies and cost savings.
For more information on how we can help your business harness the power of Generative AI for anomaly detection, visit our website at www.futureanalytica.com.